Development of a real-time fish-behaviour analysis tool for early-warning applications in aquaculturesystems (RT-FIBEAN)
DigiRAS_BCOM
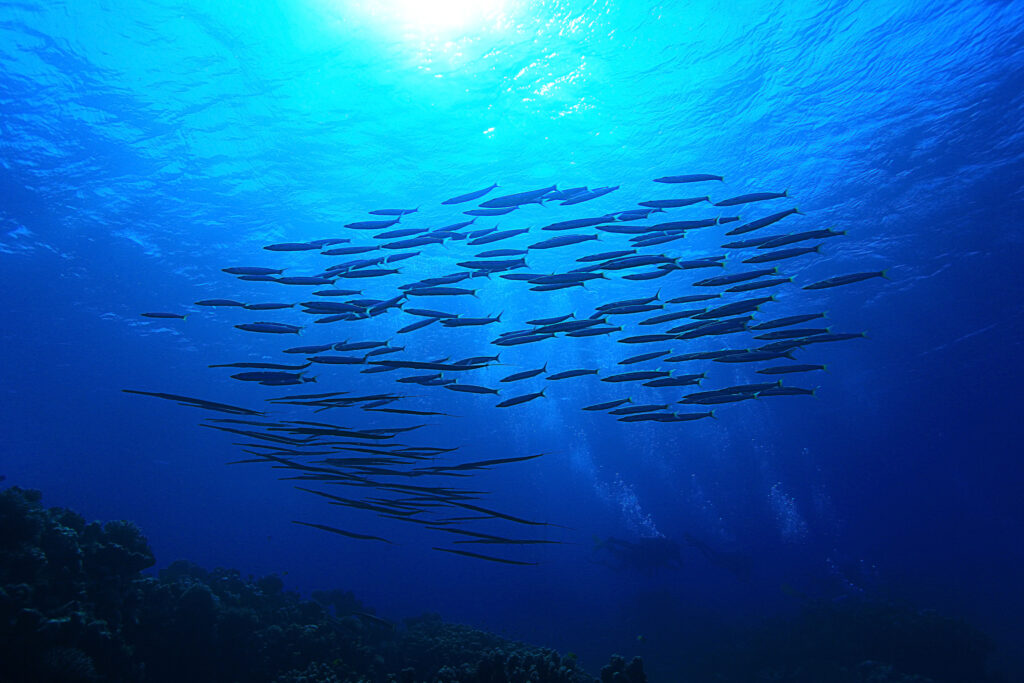
The overall aim of this research is to develop an automated system for real-time detection of changes in fish behaviour via machine vision and machine learning to reveal unfavourable environmental changes before the fish is severely harmed. We have so far developed an underwater camera system with the corresponding processing algorithms for fish behaviour tracking and obtained results after testing in demo-scale have been highly promising, including, clear data trends on detected behaviour changes of Atlantic salmon smolt in response to H2S exposure, representing a stressor with high relevance in commercial land-based aquaculture. However, with the current implementation of the data processing pipeline we are not able to achieve real-time processing of the incoming video data, which currently only allows for retrospective analyses. In order to evolve the demonstrated technology to become operational useful for the industry, real-time processing is necessary, allowing for quick decisions to maintain best possible fish welfare. Therefore, improving the developed machine learning-supported stereo camera system and processing algorithm is a necessary step towards commercialisation of the stereo camera and its processing algorithm. The current implementation of the data processing pipeline is based on an existing open-source neural network architecture, this architecture detects the region of interest of the objects. We believe that a key-point based detection algorithm will need less resources for processing and has therefore high potential to allow for real-time processing of fish behaviour video data.